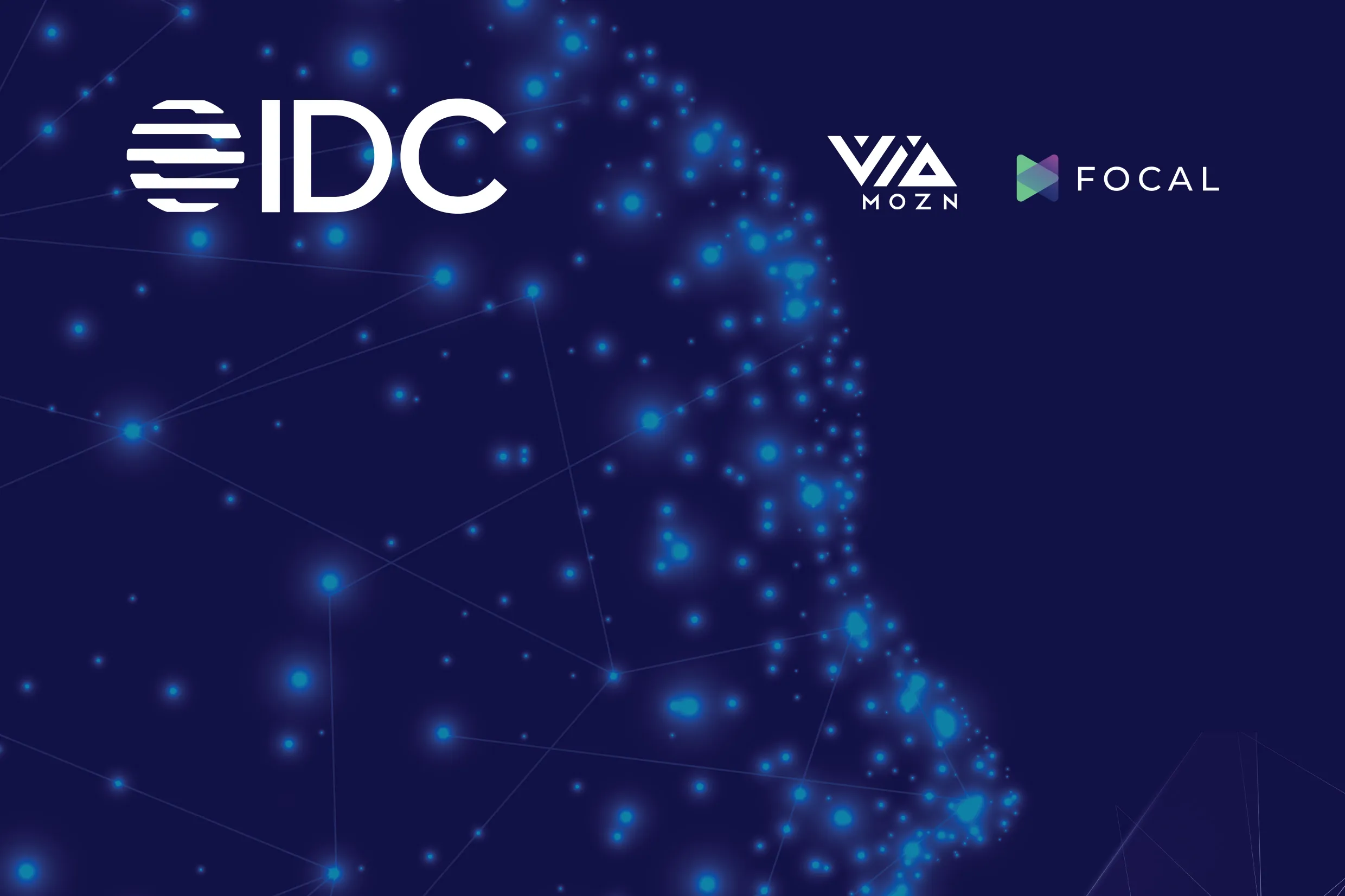
EXECUTIVE SUMMARY
Financial institutions, including banks and FinTech can respond to the increasing regulatory pressures in compliance by adopting innovative approaches using innovative artificial intelligence AML/ KYC applications to combat money laundering schemes. Using artificial intelligence will at the same time help to keep the increasing costs of compliance under control and conduct investigations with more precise outcomes.
Legacy rules-based transaction monitoring systems are becoming less efficient as the rules they monitor can be circumvented by criminals (Example) and are very labor intensive to operate and deliver too high ratio of false positive outcomes. As a result, it is cumbersome to submit a complete and actionable suspicious activity report.
Machine Learning and Natural Language Processing are two artificial intelligence technologies which can be successfully deployed to combat money laundering schemes in a cost-efficient manner. The challenges are that talent, skills and knowledge are not widespread and the algorithms and models behind the concept are difficult to understand and gain trust. Artificial intelligence use cases can be applied throughout the whole anti-money laundering end to end process from augmenting the customer due diligence process till generating well founded Suspect Activity Reports and cases with true positives with powerful analytics through efficient screening mechanisms.
Saudi Arabian financial services providers show ample demand for expanding their usage of artificial intelligence solutions and several banks are developing their road maps to augment data analytics for compliance and fraud detection. Currently, the usage of artificial intelligence is however limited and technologies like robotic process automation are used to improve straight through processing.
ARTIFICIAL INTELLIGENCE IN FINANCIAL SERVICES COMPLIANCE THE CASE TO EFFICIENTLY COMBAT FINANCIAL CRIME
It was estimated in 2009 that criminal proceeds amounted to 3.6% of global GDP, with 2.7% (or USD 1.6 trillion) are being laundered. Even though the date of publishing this report is a few years back, it would be prudent to state that there are no signs that this sum has declined since the release of the report.
As a result of this, governments have decided to pay a lot of attention to their regulatory requirements and oversight. Banks are under increasing scrutiny across all jurisdictions, and this has caused banks’ compliance costs to grow rapidly over recent years, burdening bank earnings. Banks allocate the resources to combat money laundering as regulators, such as tax authorities and regulators of financial services providers, hand out record fines to banks found guilty of anti- money laundering (AML) breaches.
To state a recent example of financial misconduct, Danske Bank was alleged that it was involved in an approximately USD 200 billion money laundering scandal. There were around 15,000 accounts under investigation which Danske Bank closed in 2015, and out of these, 6,200 were considered suspicious. The fines are estimated to be USD 3.3 billion.
A very recent but smaller, albeit equally important example, is that The United Arab Emirates’ central bank imposed financial sanctions on 11 banks operating in the country for their failure to reach appropriate levels of compliance on anti-money laundering and sanctions. The sanctions imposed on 24th January amounted to a total of 45.76 million dirhams ($12.5 million), the regulator said in a statement.
The compliance processes in money laundering have become very complex with strong labor- intensive workflows. There are multiple checks and handovers among staff and many manual processes. On top of this, there are numerous cross-country examinations and investigations, not to mention the ever-changing legal requirements which must be continuously updated and incorporated into the workflows.
And this is precisely where e.g., machine learning (also known as ML and is a branch of AI) comes into the picture, as it can analyze millions of data points to detect attempts on fraudulent schemes that would take an enormous amount of time for a human to go through. ML is also able to actively learn and calibrate itself to improve the precision and reduce in this specific case false positive cases.
ARTIFICIAL INTELLIGENCE AND COMPLIANCE DEFINITIONS & TERMINOLOGY
Customer Due Diligence / Know Your Customer (CDD / KYC)
This process is conducted with the objective to identify and verify that the applicant is who he claims to be. The applicant is also assigned a risk score based on his profile. Key steps in the process are using ML to e.g., verify the face of the applicant against an ID card or passport and potentially to conduct a screening of publicly available media content. The process for accepting legal entities is even more comprehensive as it can deal with legal documentation both in paper as well as electronic form.
The CDD / KYC procedures are indeed key and should start with intensive checks before accepting a client and continue in the form of regular reviews. The procedures should apply not only to individuals and corporations that are or may become our direct business partners, but also to people and entities that stand behind them or are indirectly linked to them, such as beneficiary owners.
Anti-Money Laundering (AML)
Anti-money laundering are measures financial services providers take to prevent and combat money laundering, terrorism financing and other financial crimes. It is conducted in accordance with the rules, regulations and processes stipulated by domestic and international law governing bodies and other organizations.
Artificial Intelligence / Machine Learning (AI / ML)
AI is usually defined as the ability of a machine to perform cognitive functions we associate with human minds, such as perceiving, reasoning, learning, interacting with the environment, problem solving, and perhaps even exercising some sort of creativity. Machine Learning is an application that provides (computer) systems the ability to automatically learn and improve from experience without being explicitly programmed. It examines patterns in the data and makes better decisions based on the examples provided (or identified by the algorithm itself), aiming to make the machines automatically learn and recreate the action without human intervention or assistance.
Natural Language Processing (NLP)
NLP is the ability to extract people, places, and things (also known as entities) as well as actions and relationships (also known as intents) and even sentiment from sentences and passages of unstructured text. It deals with programming software to process and analyze large amounts of data that has been captured in the way humans write, speak, or document information.
NLP contributes to draft the image of the client and helps to understand how clients use language and thus supports the ability to spot systemic patterns of illegal activities.
NLP is specifically useful in the context of transcribing or romanization from one alphabetic system to another or from a pictorial language through romanization to an alphabetic language. NPL takes this into consideration when spotting suspicious patters.
NPL can identify different verbal forms of the same word, which can sometimes be the same or at least very similar, like run (verb), running (adjective) and run (noun). For certain languages such as Arabic, where verbs usually consist of three root letters, morphology (the study of the forms of words) can be very important.
This technology can be very important and specific both to the CDD / KYC procedure as well as ongoing screening. Properly used it can detect suspicions and notions of financial crime when scanning articles related to suspected entities in war zones or related to corruption which may otherwise not be registered, and the connection made.
ARTIFICIAL INTELLIGENCE BASED COMPLIANCE CONCEPT THE PRECONDITIONS FOR AN EFFICIENT DEPLOYMENT
There are several technology foundations which are a prerequisite for an efficient functioning and AI deployment in compliance. These technologies should equally be in place as one technology cannot compensate for the insufficient availability or quality of another technology.
The four technologies that enable AI development are:
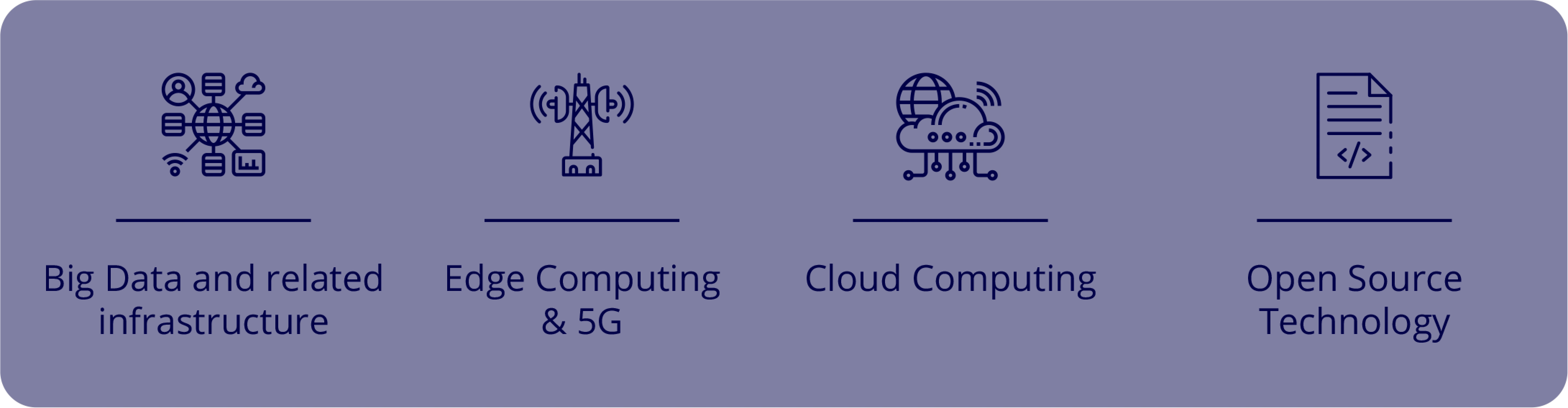
Big data infrastructure has delivered a huge boost to AI by efficiently storing and pre-processing learning data for AI learning. 5G has cut down data transmission times and made data exchange much more efficient, while edge computing allows machine learning to take place on devices like smartphones. Cloud computing has enhanced the total computing power in a revolutionary manner. Open-source technologies make contributions from AI communities and major tech giants available to all, lowering costs and enabling software innovation and collaboration.
DRIVERS FOR DEPLOYING ARTIFICIAL INTELLIGENCE SOLUTIONS
The case for changing the legacy compliance architecture is straight forward. AI algorithms can process massive amounts of data regarding many variables related to the behavior and activity information of clients and their networks. Adopting a holistic and global view the investigations are made with less resources, more efficiently and with more precise outputs, which means that the total cost of operations of the AI solutions provides a winning option. At the very end it also benefits the customers who experience a smoother and more efficient onboarding process.
Supervised machine learning can discover meaningful suspicious patterns that would not be seen with traditional (rules based) AML systems.
Drivers & Benefits – Artificial Intelligence in Financial Services Compliance
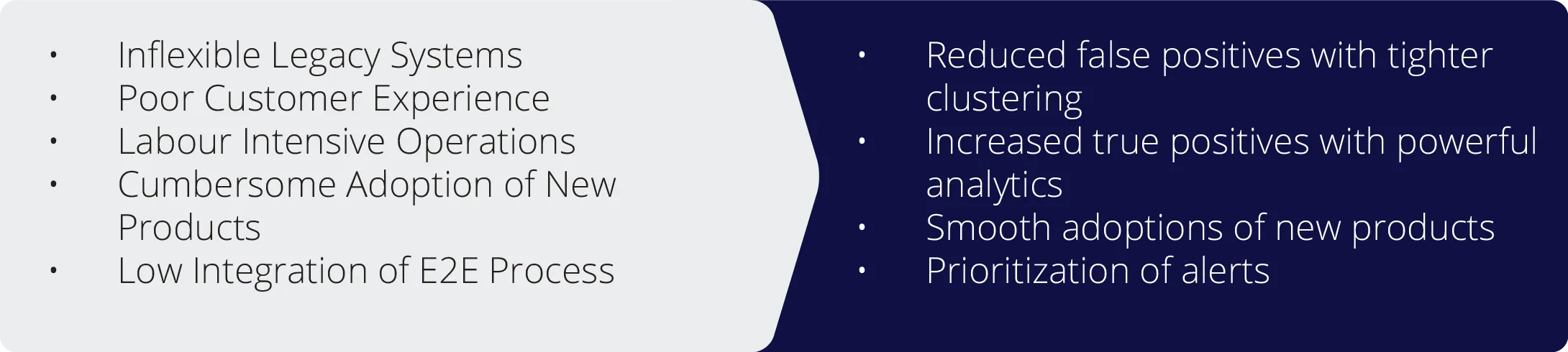
Replacing Rules-Based Legacy Systems with Artificial Intelligence / Machine Learning Algorithms
To mimic human intelligence, AI applications adopt two computation approaches: rule-based and non-rule based. Rule-based AI “learns” using pre-defined rules and knowledge, and “thinks” by inferring logical causes and effects according to “if-then-else” rules. The non-rule-based AI “learns” with machine learning algorithms and “thinks” using trained algorithm models to identify patterns of behavior.
A rules-based approach means to put in a considerable amount of effort to manage the rules engine. The number of rules can easily amount to hundreds and thousands. Further, the rules evolve and change over time, and they must be programmed into the engine.
Most traditional anti-money laundering systems are rule-based. Significant human effort is thus required to determine whether any of the transactions identified as suspicious are in fact false positives (transactions identified as suspicious but which are not) and this adds to the operational expenses.
A major constraint with the purely rules-based approach is that the engine does not “think” about developing new applications or combinations of existing rules to draw the right conclusions. Neither does it look for patterns, such as clustering of patterns representing potentially fraudulent activities.
Poor Customer Experience and Labor-Intensive Manual Work
Most AML activities require significant manual effort, making them inefficient and difficult to scale. Compliance staff must have multiple touch points with a customer to gather and verify information. In an era of mobile ordering and instant deliveries, this lengthy process falls significantly short of customer expectations. Manually verifying false alerts created during the screening cycle of the client is another example adding a manual burden.
Poor customer experience gained through the onboarding process, either physical or digital, can refrain customer from deep engagement with their financial services provider or even step away from being a customer. A remote onboarding powered by digital ID verification and supported by AI solutions can provide the client with a fluid workflow in real-time and enforce the positive image of the organization to conduct more business with it. With the implementation of AI software technologies like facial recognition and digital signatures enables real-time verification and ensures better customer CDD and KYC processes.
The customer experience also relates to the screening process after the onboarding, when unnecessary suspensions of transaction may not be undertaken, and clients may not have to be conducted to lift the suspensions.
Poor Flexibility to Incorporate New Products and Asset Classes
Common insufficiencies include that new products, such as digital peer-to-peer payments and asset classes, such as crypto currencies are typically not part of the legacy solution and must be treated manually.
Lack of Integration and Coverage of the End-to-End Process
Another insufficiency of legacy systems is that they have workflows which are not integrated. The need to conduct the initial customer due diligence (CDD) is a labor-intensive task, specifically in international banking with cross jurisdiction. Evenly important is to have a seamless integration of the initial CDD into the continuous AML monitoring. The risk scoring a clients receives based on the initial scrutiny needs to be updated and aligned with all other continuous AML screenings. Notifications must be created and acted upon when needed every time there is a major change of many factors, such as corporate governance, change in ownership, and new board members are elected in the case of legal entities.
CHALLENGES AND RISKS WITH ARTIFICIAL INTELLIGENCE IN COMPLIANCE
Even though the case for implementing AI in compliance is rather compelling, there are also certain barriers which need to be dealt with to make AI more accepted.
Lack of Ability to Explain the “Black Box”
A major inhibitor to a more widespread usage is the ability to explain the algorithms which are at the heart of the functioning of AI. This comes also with the ability to forecast the outcomes and decisions of the instructions and computations.
To gain a more widespread acceptance of AI in general requires building trust and safety mechanisms and complying with regulations through transparent, explainable algorithms.
Keeping the Regulators on the Learning Curve
The greater use of machine learning techniques to train algorithms on larger and more diverse data sets presents new complexities not only for the banks themselves but also for bank supervisors. The regulators will need to equip themselves with knowledge on data science and programming and AI in general. Further, the increased interconnectedness and growing ecosystem connections between banks, software developers / tech firms and the regulators themselves warrant ongoing monitoring of the impact of AI adoption on financial or rather operational stability of the financial services sector.
Data Privacy, Ethics & Bias
Data privacy goes hand in hand with the ability to understand and explain the functioning of AI. Clients are becoming more aware of privacy issues and have concerns about data collection and processing. A potential lack of trust is detrimental specifically to a highly regulated business as is financial services.
Particular attention should be devoted to algorithmic bias and the potential for algorithms to produce unlawful or undesired discrimination in the decisions to which the algorithms relate. Bias is in many ways built into the models and algorithms. Models are initially developed by human beings before they potentially learn to discover the biases and make them redundant themselves or rectifying them by human interaction. Another type of bias is training data only includes activity identified as suspicious in the past.
Models dealing with decision making processes may not necessarily always differentiate between causality and correlation and therefore do not offer clear explanations for a certain recommendation, e.g., why a client should be on the Suspicious Activity Report. The client may be there as the ML has learnt it from previous examples and these sub-par interpretations were made by poor human judgement.
Data Localization
Saudi Arabia’s laws around data localization are pushing organizations to keep their data stored in the country to increase security and ensure data ownership. Although, this is enabling an increase in demand for local datacenters, the challenge around data cleansing and organization remains to be unaddressed. Poor data quality can lead to inefficient decision making for the business and can increase their exposure to cyber-crime. AI and ML can provide a near accurate and flexible way to deal with improvement than conventional data-driven patterns, in turn reducing cost and improving the cybersecurity posture of the organizations.
Shortage of Talent
AI is still far from being a mature concept or widely applied technology and thus suffers from a shortage of experts. Financial services providers compete with technology companies, Fintechs, a vast array of other industries and regulators for talent.
THE CHALLENGES OF AML COMPLIANCE WITHIN A GROWING FINTECH ECOSYSTEM
Developing AI solutions always requires specialists with specific skills in data science or algorithm programming. To ensure the AI model is developed and deployed smoothly, a well-defined technology roadmap is essential, which ensures internal and external collaborations are properly considered and managed.
As with any other technology the eternal question of buy vs build comes up. There is several global technology players catering for AI based solutions as well as local developers who may have the right resources in place to provide tailor made solutions for specific needs.
It was stated earlier that data is essential for the proper learning process of the AI solution and data sources can be sourced either internally within the bank or through collaboration with an external partner. In any case, this poses certain risks as to the quality and validity of the data.
Another point to consider is the data access controls of the vendor or partner before the banks incorporates the solution into their own landscape. A major risk, as with any other dependency, is that the vendor will not fully understand the needs of the user. The learning and calibrating of the AI solution or rather the ecosystem of interactive solutions must be constantly upgraded to allow for the discovery of new patterns and emergence of new entities.
Open banking and the development of multilayered APIs present a risk at the same time as an opportunity to perform KYC / AML screenings by accessing and evaluating customer journeys and behaviors. NLP can play a significant role here as it does not limit itself to one single ecosystem. The open-source ecosystem widens the adoption of practical use cases, which can be a useful reference point for banks wishing to improve existing models that they have developed in-house.
ARTIFICIAL INTELLIGENCE / MACHINE LEARNING IN PRACTICE SELECTED USE CASES
The approach to deploy AI / ML varies dependent on the maturity of the financial services provider but in essence there are two major directions:
a) Use AI/ML for all types of KYC / AML related activities which in most cases means to replace manual and / or technology supported rules-based engines. This is applicable in the case the financial services provider decides to go for a big-bang replacement or in case of building the compliance as a green-field operation.
b) Adopt a combination of machine learning as a technology support system to interact with the existing rules-based engine. This will add value to existing processes in areas, such as risk rankings / ratings and prioritize alerts. AI / ML can also support the process of adjusting and adapting the rules to be able to spot criminal or fraudulent transactions. This is currently the most common approach.
Customer Onboarding Phase
Facial & Document Recognition
A remote account opening, as opposed to the in-person account opening, involves a different workflow for client identification. A crucial part is to extract ID information with machine learning enhancing the scanning through Optical Character Recognition (OCR).
ML is able to e.g., verify applicants’ identity using facial recognition and biometric liveness detection. Facial recognition technology can match the applicant’s face with that of the photo extracted from their ID document. ML supports this step by detecting if e.g., the variances between the two pictures are within a tolerance zone, or if the face is a real face or a mask. The applicant can be asked to take multiple digital images at different angles to verify unique security features on the ID card, such as microprint, hologram, layout, and font. The applicant may also be asked to record a short selfie video, and a face extraction model is used to verify whether the video was shot by the user in a live environment. ML helps to enhance OCR technology by typically makes use of neural network models that can deal with complex backgrounds, noise, lighting, fonts and other distractions.
ML can also help to verify if the scanned ID card itself is fake. However, to do the actual enquiry into a database to check if the ID card or passport is stolen is to be done by a less sophisticated system.
Risk Scoring
Risk scoring in connection with the CDD has the important function of detecting suspicious applicants in the very beginning of their customer journeys with the bank. Further on, the risk scoring will also be updated based on the client’s behavior.
The ML algorithm will assign risk score to applicants based on several criteria or values or status of input data. Examples of this is citizenship, residency and permanent address including postal code, ownership of property or leasing of the applicants dwelling, etc.
Customer Transacting Phase
Risk Scoring
The risk scoring is an ongoing activity after completion of the initial CDD / KYC onboarding process. Data about the customers transactional pattern are evaluated by the ML algorithm. This approach can be enriched by combining external data to give a fuller picture in the continuous KYC customer process. This historical client behavior can include various anomalies and suspicious activities that result in filing an SAR internally for further investigation or directly to the regulator. The risk rating can also be inferred to put a risk score on other customers with similar behavior and investigate if there are similar patterns and attributes and understand correlations. The ML models are trained for this type of ongoing customer screening.
Clustering of Clients to Spot Suspicious and Fraudulent Behavior
The basic purpose of creating client clusters is to discover hidden patterns of similar transactional behavior and these patterns are set as the baseline for normal transactions. Thereby is it possible for the unsupervised machine learning clustering algorithms to discover clusters of transactions which can be suspicious or directly criminal in its nature.
Anomalies, or statistically speaking outliers, which lack strong membership to any cluster group, can be subject to additional review by human staff for confirmation of their legitimacy. If this is the case, then these results can be fed back to the learning model to improve accuracy through reinforcement learning.
As a result, the false positive rate for flagging laundering risk can be substantially reduced by the consolidated ML solution. Another benefit is the ability to cluster clients in a significant manner provides valuable inputs for the risk scoring function.
AML Screening and Predictions
Traditionally, banks use rule-based systems with predefined transaction patterns to identify potential money laundering activities. In cases of complex transactional behavior and patterns, the volume of false positive alerts identified by this approach increases, requiring significant time to investigate alerts and the need to filter false alarms.
ML models, trained by customer and merchant data from various internal and external data sources, are used to understand business activities and complex transactions. The trained models can be deployed to scan through all the suspicious transactions identified by the AML solution to differentiate false positive alerts from transactions that really require investigation. To continuously improve the model, new data patterns and the results of investigations by humans on identified suspicious transactions are looped back to the ML algorithm model for more reliable future recommendations. By combining both supervised and unsupervised learning models, detailed individual customer risk profiles can be built. Every transaction can then be scored based on the corresponding level of fraud or money laundering risk. Such risk prediction input has been widely adopted to enhance existing AML decision engine rules in use cases such as e-commerce transactions, mobile banking, loan applications and recurring banking payments.
Natural Language Processing – Unstructured Text Screening
Social media posts and an individual’s digital footprint can be used to assess the risk profile of potential customers. But the text analysis of unstructured data can be used to screen fraudulent emails to spot a common syntax of the language, identify names of natural or legal entities, check the spelling, etc. to cluster the text based on topics, context, and other parameters. This screening can be enhanced with high-quality real-time global watchlist data.
STATE OF ARTIFICIAL INTELLIGENCE IN COMPLIANCE IN SAUDI ARABIA
Saudi Arabian financial services providers have in general a solid base understanding of artificial intelligence and its subsets, such as machine learning. They apply chatbots providing basic information and as a second step plan to introduce transactional support. But still a lot of focus is on automatizing processes through robotic process automation than deploying AI models and algorithms. Compliance operations and regulatory requirements are mainly supported by rules- based solutions and intensive human interaction.
To remedy this situation Saudi Arabian financial services providers, show ample demand for expanding their usage of AI solutions. They are setting up centers of excellence to accumulate knowledge and skills to be able to prepare for the future.
Many institutions have AI on the road map both in terms of adopting it for regulatory purposes, such as compliance and fraud detection, but also for improving the customer experience to generate better sales propositions.
One of the major challenges to move fast on the learning curve is the scarce supply of talent and practical skills. Financial service providers are prospecting partners to implement the AI solutions with and to build up internal capabilities.
The Saudi Arabian regulator has recently introduced an open banking policy which will put financial services providers under pressure to stay competitive but will also indirectly force them to re- consider their compliance processes given the vast amount of data which will be made available to them.
ABOUT MOZN
Mozn was established in 2017 in response to an increasing demand for data dependent decision making across sectors. Mozn’s team is built around the latest in digital technologies i.e., AI, ML and BDA. This enables Mozn to deliver impact through data, anytime, anywhere. Mozn is now the market leader in building and deploying bespoke advanced analytics solutions in the Middle East, and developing data and analytics driven products in niche areas globally.
Through our proprietary R&D in Risk and NLU, SaaS AI Products and AI Solutions, we transform the way our clients work and generate long-lasting business impact. The aim of the company is to empower enterprises in the region to make mission-critical decisions through the power of data.
FOCAL: AML SUITE & SANCTIONS SCREENING
Amongst a wide range of business solutions, Mozn’s Focal for banking, finance, securities, and insurance helps these organizations to become more compliant with AML regulations and reduce risk of financial crime without the complexity of screening and integration. It is a sanctions screening solution powered by a proprietary AI-enabled engine for Arabic and Latin names to help MENA organizations eliminate false negatives, reduce false positives, and increase confidence in their screening efforts.
- Focal is the first web-based screening tool that is locally hosted in the region to be compliant with local cybersecurity regulations.
- With Focal, Mozn offers a unique value to MENA’s fintechs in terms application integration and local cloud hosting with access to a global database of +1000 sanctions lists, PEPs, and adverse media.
- Focal is becoming a one-stop-shop for all AML compliance needs beyond AML screening, such as transaction monitoring and risk scoring.
- Focal’s mission is to dismantle AML compliance barriers and accelerate the deployment of advanced infrastructure geared to tackle financial crime using state-of- the-art technology and simple-to-use software, bringing MENA’s fintech ecosystem to the global financial stage.
CONCLUSIONS & RECOMMENDATIONS
The accelerating development in big data and analytics, as well as the progress in the other preconditional technologies and hardware, will surely make AI a more integral part of decision making and give the AML officers a stronger weapon to combat fraudulent behavior.
- Artificial intelligence, such as machine learning models can change the architecture of AML. This is possible thanks to the availability of new technologies, such as big data analytics and cloud computing.
- The gradual shift from a rules-based engine to a machine learning approach will fundamentally transform the compliance function. The impacts will be significant in terms of achieving more precise and relevant outputs while at the same time be more efficient.
- There are inevitably several risks with the transition. Many perceive AI as a “black box” where even its operators cannot explain why the solution came up with a specific decision.
Financial services providers should:
- Consider adopting tools with AI-enabled engine for Arabic and Latin names to eliminate false negatives, reduce false positives, and increase confidence in their screening efforts.
- Consider options for the target AML architecture dependent on the starting point and level of maturity. In case of starting with a rules-based legacy system to consider a hybrid model of a rules-based solution supported with machine learning model.
- Get onboard necessary talent with a blend of deep skills in statistics, data analytics and technology.
- Define the major use cases and initially conduct a simplification of the underlying processes. Start small by selecting a model. Then train and recalibrate it in a closed environment.
- Search for practical partnerships with external consultants and technology vendors to develop in-house capabilities.
About IDC
International Data Corporation (IDC) is the premier global provider of market intelligence, advisory services, and events for the information technology, telecommunications and consumer technology markets. IDC helps IT professionals, business executives, and the investment community make fact-based decisions on technology purchases and business strategy. More than 1,100 IDC analysts provide global, regional, and local expertise on technology and industry opportunities and trends in over 110 countries worldwide. For 50 years, IDC has provided strategic insights to help our clients achieve their key business objectives. IDC is a subsidiary of IDG, the world’s leading technology media, research, and events company.