
The Status Quo and the Challenge
Companies, organizations, and governmental institutions in the Kingdom of Saudi Arabia and all over the Arab world all keep immense quantities of documents written in the Arabic language. Such records and documents include unstructured data existing in the form of email correspondences, excel sheets, digital reports…etc. The data, although unstructured, holds tremendous value for companies, organizations, and institutions. Insights, if properly generated, can add value to executive, strategic, and operational goals and assessment of KPIs.
Unstructured data exists in large quantities, yes. However it's a challenge to delegate the task of data analysis – especially unstructured data – to human analysts because the task is time-consuming and costly. Furthermore, human analysis is unreliable; it does not guarantee arriving at results or generating valuable insights consistently in such a way that benefits the goals of the respective organization.
Natural Language Understanding Technologies (Today)
The field of Natural Language Understanding (NLU) and associated technological techniques evolved to achieve this complex analytical task. However, the task remains a challenge even for State-Of-The-Art (SOTA) technologies that fail to achieve significant performance when it comes to documents and records intelligence.
One of the most powerful analytical NLU techniques is Named-Entity-Recognition (NER). NER is an NLU technique created to identify key information in unstructured textual data. It classifies the information (Entities) identified to different categories (Entity Types) and thereby structures the inputted data and paves the way for extracting relationships and hidden insights in the large quantities of unstructured textual data. In essence, NER is one of the most powerful techniques that enable the generation of valuable insights from textual information in documents and records.
NER engines are computational models built to absorb unlimited quantities of textual information from a variety of sources and output the identified entities and entity-types. Given the complexity of the NER NLU technique, up till now, there hasn’t been any NER engine sophisticated enough to generate insights up to and even better than a human-level performance.
In this blog, we present Mozn’s Arabic-first NER engine and showcase its record-breaking performance - the industrial and academic leader of the machines specialized for Arabic Language Intelligence.
Obstacles
So what are the obstacles standing in the way of analyzing Arabic texts and generating valuable insights?
First: Weak or non-existent Data Structuring:
80% of the data that organizations hold exists if at all in an unstructured and unorganized format that's improperly archived if archived at all. [1]
Second: Limitations of SOTA Arabic text NLU technologies.
The majority of the NLU technologies that analyze Arabic texts only do so superficially. Consequently, their ability in analyzing Arabic texts and generating insights is limited.
Responsibility
Mozn is on a mission to change this status quo by transforming the process of searching to an intelligent and powerful one especially with respect to its insights-generation capabilities.
The Choice
To do justice to the Arabic language, Mozn followed a strategy centered around building an advanced NER engine that intelligently identifies various patterns and insights from Arabic texts in such a way that parallels human-level performance. To ensure achieving the highest standards of performance, Mozn invested in top Arab talent to lead the research and development of the language intelligence models.
The Mozn Way
Furthermore, Mozn took into consideration the time and place factors in determining the types of entities that would be most valuable for regional organizations and governmental institutions.
Effectively, Mozn has built a NER engine capable of identifying more than 22 types of entities, a number greater than that of OneNotes, one of the most well-known datasets in the field.
Why NER
Entity recognition enables the generation of insights from unstructured data represented by texts existing in various forms written in a variety of styles and reflecting multitudes of contexts. This technique is of particular regional significance. Each country in the Middle East has its own unique nuanced uses of the Arabic language and certain Arabic entity types in particular. For instance, the entities “Minister” and “King'' are mentioned significantly in the majority of official documents and media news in the GCC. These titles and associated names also reflect on some of the names of institutional authorities, organizations, or even public facilities. Therefore, capturing the contextual nuances of Arabic language is crucial for proper Arabic language intelligence and insights generation.
Quality recognition has yet to be achieved for the Arabic language by the SOTA global engines. Evidently, when examining the performance of the different NER engines in recognizing entities within Arabic texts as shown in Figures 1-2, we find that global NER engines fail significantly in comparison to Mozn’s NER engine when it comes to capturing the nuanced complexity of the Arabic language for the local context. We take the following example (Figures 1-2) to showcase the limitations of the SOTA global engines in capturing the complexity and nuances of the Arabic language in a local context.

Figure 1. This NER engine only manages to identify the name of a person without capturing its reference to the facility. Further, this NER engine does not identify any additional entities beyond the person.

Figure 2. Mozn’s NER engine on the other hand, besides identifying more entities than global competitors, manages to identify the facility appropriately as a facility and does not confuse the entity with the person if a person’s name is associated. Clearly, Mozn’s NER engine excels by far in capturing complexity in Arabic texts and allows for enhanced capabilities in language intelligence.
Comparative Benchmarking
Mozn didn’t stop at identifying its objectives directed towards transforming the Arabic text search process; It showed and achieved exceptional results reflected by the performance of its NER engine, outcompeting all global academic and commercial competitors in the specific contexts for which its NER engine was developed for. We showcase the results below:
- On the Commercial Competition Front:
The following is a comparison between the performance of Mozn’s NER engine and that of top global players
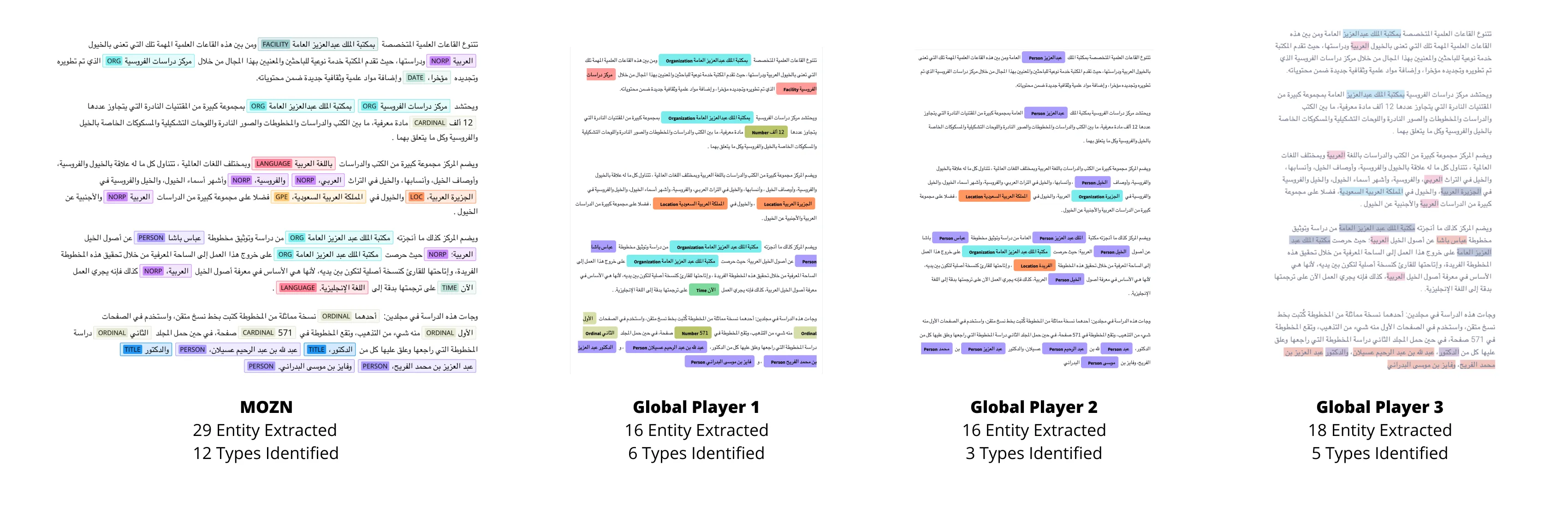
Figure 3. NER results comparison of Mozn and Global Players. All engines received the same text. Mozn NER engine excelled by extracting the greatest number of entities (29) and entity types (12).
- On the Academic Competition Front:
Mozn’s NER engine performance was also compared with the State-of-the-art (SOTA) engines developed by top academic institutions. As shown below, the results achieved are outstanding by global standards.

Figure 4. F1 Score is a standard measure of accuracy that is the average for precision and recall. Mozn’s Arabic-first NLU engine achieved a higher F1 score than the SOTA Arabic NLU NER engines in academia, and has proudly become the first arabic-first NLU engine to come close to SOTA English-first NLU NER engines.
Leadership
From this outlook, and notwithstanding the exceptional results achieved, Mozn is proud to be the leader in its Arabic-first NLU NER Engine and Arabic language intelligence capabilities. This leadership allows Mozn to continue to lead in further capabilities such as relationships extraction, text summarizations,and question answering. Collectively, Mozn is set to lead in providing top Arabic-first NLU capabilities for insights-generation from Arabic documents and records.
Destination
Having a quality NER engine to build on additional language intelligence capabilities is paramount to all subsequent milestones towards building the sought after insight engines. Mozn is looking forward to continuing to augment its technological capabilities for insights generation to help the regional technological infrastructure and raise the standards of the Arabic language NLU globally.
[1] 80 Percent of Your Data Will Be Unstructured in Five Years (solutionsreview.com)